Kindness as a KPI
Leveraging empathy and measuring the unmeasurable.
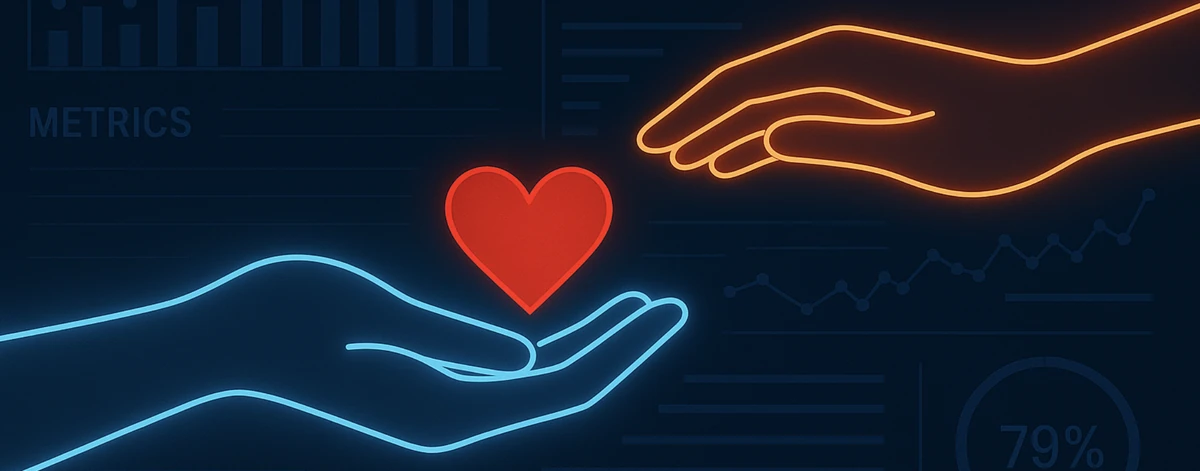
In a world obsessed with hard metrics—conversion rates, churn percentages, and quarterly profits—some companies have discovered the unmistakable power of something far less tangible: kindness. Not as a vague corporate value buried in an employee handbook, but as an actual measured key performance indicator driving strategic decisions and brand identity.
The story that sparked this reflection involves pet supply retailer Chewy and how they respond when customers lose their beloved pets. When Anna Brose contacted Chewy in June 2022 to return unopened dog food after her dog Gus died, the company didn’t just process a routine return. Instead, they refunded her purchase, suggested she donate the food to a local shelter, and then—most remarkably—sent flowers with a handwritten note of condolence. Her tweet about this experience went viral with over 680,000 likes and 40,000 retweets (Newsweek, 2022).
This wasn’t an isolated incident or the work of a rogue customer service agent. It’s a long-standing company practice dating back to at least 2017, part of a deliberate strategy where empathy isn’t just encouraged—it’s measured, incentivized, and celebrated.
When Kindness Drives Numbers
The business impact of these “kindness metrics” defies conventional wisdom about return on investment. According to Forrester Research, “Devoted customers will pay 50% to 200% more to stay with your brand” when you inspire and maintain their loyalty through exceptional customer experience.
For Chewy, this approach has translated to astounding loyalty metrics. Their compassionate approach to customer service has created countless loyal customers who publicly declare they’re “customers for life” after experiencing Chewy’s empathy firsthand.
The Ripple Effect
What makes kindness metrics particularly powerful is their ripple effect. When Southwest Airlines tracks and rewards employees for “Proactive Customer Service Events” (unexpected acts of assistance beyond formal requirements), they’re measuring a leading indicator that predicts everything from customer satisfaction to social media sentiment.
Research published in the Harvard Business Review demonstrates that customers who have the best past experiences spend significantly more compared to those who had poor experiences. Companies that invest in customer experience see measurable financial benefits that extend far beyond the immediate transaction.
The concept extends beyond customer interactions. At Patagonia, executives measure employee volunteer hours and environmental impact alongside financial performance, recognizing that these kindness metrics ultimately strengthen their brand identity and customer loyalty in measurable ways.
Kindness is deeply rooted in the company culture and in turning everyday interactions into extraordinary experiences.
Finding Your Kindness Metric
The challenge for leaders isn’t whether to measure kindness, but how. Some approaches that work:
- Track and celebrate stories of exceptional employee empathy
- Measure problem resolution satisfaction, not just time-to-close
- Survey customers about emotional connection, not just transactional satisfaction
- Create space for employees to act with autonomy when empathy demands it
Perhaps the most important measure is the simplest: Would your customers tell a story about your kindness to someone else? In a world where differentiation is increasingly difficult, the companies that make kindness measurable are discovering that empathy might be the most undervalued asset on their balance sheet.
When we measure what matters most to humans—not just as consumers but as people—we build businesses that matter.
Share this post
Twitter
Facebook
Reddit
LinkedIn
Pinterest
Email