Ethics by Design: Embedding Responsible AI Principles
A practical approach to integrating ethical considerations throughout the AI product development lifecycle.
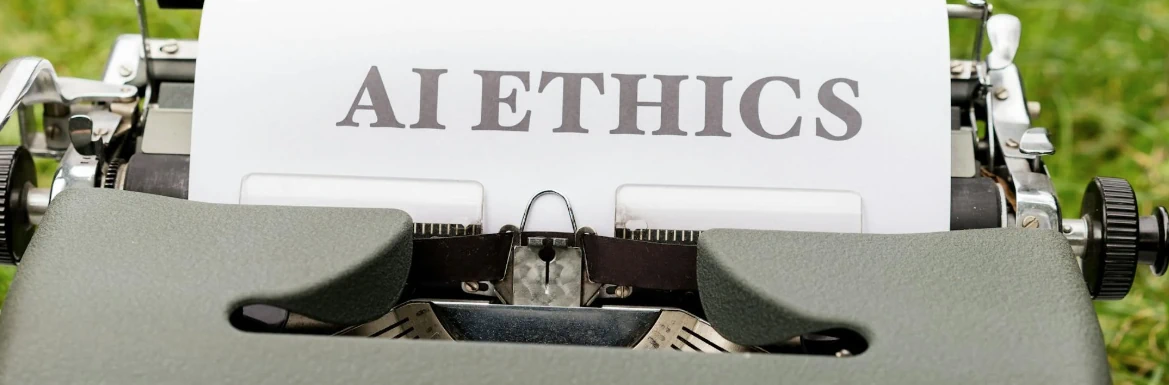
Ethics as a Competitive Advantage
The era of “move fast and break things” is giving way to a more thoughtful approach to technology development—particularly when it comes to artificial intelligence. As AI systems become integrated into the critical aspects of our lives, the ethical implications of these technologies can no longer be treated as an afterthought or relegated to a long-forgotten compliance checklist.
For product teams building AI-powered solutions, ethics isn’t just about avoiding harm—-it’s about creating better, more sustainable products that users can genuinely trust. Trust and confidence are frontline features. According to Transcend.io’s research, explainable and ethical AI implementation has become a business necessity rather than an academic curiosity as consumers increasingly demand transparency and fairness from their products.
But how do we move from broad ethical principles to practical implementation? How do we ensure that they don’t simply become a box-ticking exercises tacked on at the end of a project, but rather an integral part of the development process from the very beginning?
Beyond High-Level Principles
Many organizations have published AI ethics principles, from Google’s well-known AI Principles to ISO’s efforts to standardize responsible AI practices. While these high-level guidelines provide valuable direction, translating them into actionable steps throughout the product development lifecycle remains challenging and the bane of most company’s legal and InfoSec teams.
The gap between abstract principles and practical implementation is where many AI ethics initiatives falter. As researchers from Springer Link noted in 2023, ethics frameworks too often remain conceptual, without clear pathways to integrate them into the actual development process.
The concept of “Ethics by Design” offers a solution to this challenge—embedding ethical considerations into every phase of AI product development, similar to how “Privacy by Design” has become standard practice in data protection. Let’s explore what this looks like in practice.
A Framework for Ethics by Design
Implementing Ethics by Design means systematically addressing ethical considerations throughout the AI product lifecycle. Here’s a practical framework based on research and industry best practices:
1. Planning and Problem Definition
Before a single line of code is written, ethical considerations should be front and center:
- Ethical Impact Assessment: Conduct an initial assessment of how the proposed AI system might affect different stakeholders, including potential benefits and harms.
- Define Ethical Boundaries: Establish clear ethical guardrails for the project, identifying use cases or applications you explicitly will not pursue.
- Stakeholder Mapping: Identify all groups who might be affected by your AI system, paying particular attention to vulnerable populations who might not typically be represented in your user base.
- Purpose Definition: Clearly articulate the purpose of the AI system, ensuring it aligns with responsible innovation goals rather than merely pursuing capability for its own sake.
2. Data Collection and Processing
The ethical quality of an AI system is largely determined by its foundational data, training sets, and corpus of knowledge:
- Representative Data: Ensure your training data includes diverse perspectives and adequately represents all groups your system will affect.
- Consent and Rights: Verify that all data was ethically sourced with appropriate consent and usage rights.
- Bias Identification: Proactively examine your datasets for historical biases that could be perpetuated or amplified by your AI system.
- Data Minimization: Collect only what’s necessary, in line with privacy-preserving principles.
3. Model Development and Testing
As you build and refine your models, embed ethics throughout the process:
- Interpretable Design: Prioritize model architectures that allow for appropriate levels of explanation and transparency.
- Fairness Metrics: Implement concrete metrics to evaluate fairness across different demographic groups.
- Adversarial Testing: Stress-test your models with challenging scenarios designed to uncover potential ethical issues.
- Documentation: Maintain thorough documentation of model choices, limitations, and performance characteristics across different populations.
4. Deployment and Monitoring
Ethical responsibility extends well beyond initial deployment, but to deployment, monitoring, and iterative improvement:
- Gradual Rollout: Consider a phased or pilot deployment to identify issues in controlled environments before wide release.
- Monitoring Systems: Implement robust instrumentation and monitoring for unexpected behavior, performance degradation, or emerging biases.
- Feedback Channels: Create accessible mechanisms for users to report concerns or unexpected system behaviors.
- Update Protocols: Establish clear processes for addressing issues that emerge post-deployment.
5. Governance and Accountability
Sustaining ethical AI requires appropriate governance structures:
- Clear Ownership: Designate specific individuals responsible for ethical oversight throughout the AI lifecycle.
- Cross-Functional Input: Ensure diverse perspectives beyond just technical teams, including legal, policy, and domain experts.
- Continuous Education: Invest in ongoing ethics training for all team members involved in AI development.
- Review Processes: Implement regular ethics reviews at key development milestones.
Real-World Implementation Strategies
Moving from framework to implementation requires practical strategies that product teams can adopt:
1. Ethics Champions
Designate “ethics champions” within product teams who have specific responsibility for raising ethical considerations throughout the development process. These individuals should receive specialized training and have clear authority to flag potential issues.
IBM’s approach to implementing trustworthy AI illustrates this strategy well, as they have developed dedicated roles and governance frameworks for ethical oversight, as documented in their case study.
2. Ethics Design Sprints
Incorporate dedicated “ethics design sprints” at key phases of development, bringing together cross-functional teams to identify and address potential ethical issues. These focused sessions can help surface concerns that might otherwise go unnoticed in the regular flow of development.
3. Documentation and Artifacts
Create practical templates and artifacts that make ethical considerations concrete, such as:
- Ethical risk registers
- Fairness testing reports
- Model transparency documentation
- Governance accountability matrices
These artifacts ensure that ethical considerations are explicitly discussed and documented rather than remaining implicit assumptions.
4. Pre-Mortem Analysis
Before major development phases or releases, conduct “ethical pre-mortems” where team members imagine potential ethical failures and work backward to identify preventive measures. This approach helps teams anticipate problems rather than merely reacting to them.
5. Ethical OKRs and Metrics
What gets measured gets managed. Include specific ethical objectives and key results (OKRs) alongside traditional product metrics. This might include measurements of:
- Fairness across demographic groups
- Transparency and explainability
- User trust and perception
- Incident response time for ethical issues
Case Study: Applying Ethics by Design
Consider a hypothetical company developing an AI-powered hiring tool to screen job candidates. Here’s how they might apply Ethics by Design throughout their development process:
Planning Phase: The team conducts an ethical impact assessment, identifying potential risks including bias against underrepresented groups and privacy concerns with candidate data. They establish clear boundaries, deciding not to implement facial analysis or accent detection features due to documented fairness issues.
Data Phase: They carefully audit their training data, discovering an underrepresentation of certain demographic groups. They implement a data enrichment strategy to ensure more representative training data, while establishing strict data governance policies for candidate information.
Development Phase: The team selects model architectures that allow for interpretability, enabling them to explain how different factors influence recommendations. They implement multiple fairness metrics to assess model performance across different demographic groups, and discover concerning performance disparities for candidates with employment gaps.
Testing Phase: Through adversarial testing, they identify that the system penalizes candidates who use certain culturally-specific language patterns. This prompts model refinement and additional fairness constraints before deployment.
Deployment Phase: They implement a phased rollout, first deploying the system with human oversight where hiring managers review all AI recommendations. Their monitoring system flags unexpected patterns in recommendations for certain job categories, prompting immediate investigation.
Governance: The company establishes a cross-functional AI ethics committee that reviews key development decisions and performance metrics quarterly, with authority to require changes if ethical concerns emerge.
Building a Culture of Ethical Innovation
Ultimately, successful Ethics by Design isn’t just about frameworks and processes—it’s about culture. Organizations that excel at ethical AI development foster environments where:
- Team members feel empowered to raise ethical concerns without fear of slowing down development
- Diverse perspectives are actively sought and valued in design and development decisions
- Ethical considerations are seen as enhancing product quality rather than constraining innovation
- Learning from ethical challenges is celebrated rather than hidden
As tech-stack.com notes, the ethical use of AI isn’t just about avoiding harm—it’s about building sustainable technological ecosystems that create long-term value.
The Path Forward
As AI capabilities continue to advance, the imperative for Ethics by Design will only grow stronger. Organizations that embed ethical considerations throughout their product development will not only mitigate risks but build more trustworthy, sustainable, and ultimately successful products.
The question isn’t whether to implement Ethics by Design, but how quickly and effectively we can make it the standard approach for AI development across the industry.
For product leaders, the message is clear: ethics isn’t a constraint on innovation—it’s a catalyst for building AI systems that truly serve human needs and values.
By designing with ethics from the start, we build products that don’t just work well, but work well for everyone.
Share this post
Twitter
Facebook
Reddit
LinkedIn
Pinterest
Email